In the context of digital onboarding, demographic features such as ethnicity, age, gender, socioeconomic circumstances, and even camera/device quality might affect the software’s capacity to match one face to a database of faces i.e. AI Bias. The quality and resilience of the underlying database in various sorts of surveillance might feed bias in the AI models. Biometrics are used in modern face recognition software to map facial traits from an image or video. The information is then compared to a database of known faces to identify a match.
However, facial recognition and facial authentication have two very different processes.
The face authentication AI algorithms in identity verification are used to compare a customer’s selfie to the photo on their identification document, and bias may sneak into algorithms in a variety of ways. Even when sensitive factors like gender, color, or sexual orientation are excluded, AI systems learn to make conclusions based on training data, which might contain biased human decisions or reflect historical or societal imbalances.
What are the five essential questions to ask solution providers to assess their ability to mitigate AI bias?
How large and diverse is your training database?
Machine learning models employ AI training datasets to learn how to spot patterns and use technologies such as neural networks so that when provided with new data in real-world applications, the models can make correct predictions. When it comes to artificial intelligence, size counts. The training data set’s capacity to survive the introduction of AI bias improves with size and representativeness.
Where did the data for the training data sets originate from?
When a company’s data is insufficient to develop credible models, it typically turns to third-party data sources to fill the void, and these acquired datasets might add inadvertent bias. A collection of photographs of ID papers acquired in ideal lighting circumstances with high-resolution cameras, for example, is not typical of ID images captured in the actual world. Unsurprisingly, AI models based on unrealistic models will struggle with IDs acquired in low-light conditions. Algorithms designed using real-world production data, on the other hand, will include documents with real-world flaws. As a result, these AI models are more stable and resistant to demographic bias.
What labels were assigned to the data sets?
In most AI projects, classifying and labelling data sets takes some time, especially if the accuracy and granularity are high enough to fulfil market expectations. Labelling is how ID papers are labelled in the context of identity verification. If the photo on the ID has been tampered with, the document will be marked as fake with photo tampering. If the ID’s image has a high glare, the label should indicate this. If incorrect labels are used when labelling individual identity verification transactions, the AI models will incorporate that information into the algorithms, making the models less accurate and more prone to bias.
Some solution providers outsource or crowdsource the tagging exercise, whilst others insource it to skilled agents who are trained on how to tag verification transactions in order to maximize the AI models’ learning curve. Insourcing models, by definition, provide more accurate models.
What quality controls are in place to oversee the tagging process?
Unfortunately, most of this prejudice is unintentional since many solution providers do not realize when they create the algorithm that it may produce inaccurate results. That is why quality control must be incorporated into the process. There is no replacement for having a skilled team of tagging specialists who know how to correctly tag individual ID transactions and auditing systems in place to check their work in the identity verification sector.
How diversified is the algorithm development team?
Reducing bias also involves the individuals who create the AI algorithms and tag the datasets. It is not unjust to inquire about the AI team’s makeup. AI engineers and data scientists should ideally come from a diverse range of nations, genders, races, career experiences, and academic backgrounds. This variety ensures that many viewpoints are brought to bear on the models being developed, which can help decrease some demographic bias.
There is a growing worry that AI bias in a vendor’s AI models might harm a company’s reputation and perhaps lead to legal concerns, especially when economic choices rely on the accuracy and dependability of such algorithms. Believe it or not, these algorithms may lead to some sorts of clients being unfairly rejected or devalued, resulting in lost business and downstream prospects. That is why it is becoming increasingly crucial to understand how suppliers evaluate AI bias and what steps they are doing to address it.
Learn more about IDcentral’s Identity Verification Solution with AI Based Face Identification
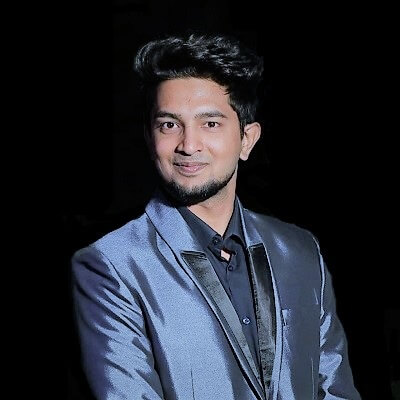
Sumanth Kumar is a Marketing Associate at IDcentral (A Subex Company). With hands-on experience with all of IDcentral’s KYC and Onboarding Technology, he loves to create indispensable digital content about the trends in User Onboarding across multiple industries.